Analyzing a Simplified Airborne Infection Model with FATE Nuclear Safety Software
By Bob Apthorpe, Senior Nuclear Engineer, Fauske & Associates
The FATE™ code is a versatile program for analyzing the transient behavior of facilities and systems during normal and off-normal conditions. It has traditionally been used for modeling fire and smoke transport, hydrogen production and migration, as well as predicting pressure and temperature behavior of nuclear waste during packaging, drying, transport, and storage. A unique feature of FATE is its ability to characterize and track aerosols including deposition from settling, impaction, and filtration.
To illustrate this capability, I'm going to develop a simplistic model of a doctor's office waiting room containing two patients: one coughing infected patient (Marty Measles) and one healthy patient (Fiona Fitfiddle). Our goal is to estimate the chance of infection due to inhalation of an airborne pathogen, in this case viruses shed by the infected patient and released as an aerosol to the waiting room environment.
Important note and disclaimer: This model is for informational use only. I am not an infectious disease specialist, epidemiologist, or industrial hygienist, and this model has not been reviewed by one. I've made a "best-effort" attempt to use reasonable parameter values and an appropriate dose model but the results are only intended to show the behavior of the FATE code, not as guidance on preventing any disease. Suggestions for improvement are cheerfully encouraged.
Also note that this model is absolutely worthless for analyzing diseases like Ebola which don't spread via inhalation. According to the US Center for Disease Control, the Ebola virus does not spread through the air. This model is more appropriate for modeling diseases which spread through inhalation such as whooping cough (pertussis), measles (rubeola), tuberculosis, or influenza.
With that disclaimer out of the way, let's start breaking down this problem into logical chunks.
Modeling the Waiting Room and Ventilation System
Let's start with the easiest part of the model to describe - the waiting room and its ventilation system.
Not-a-Doctor Bob's Clinic for the Occasionally Irritated has a 20-foot-square waiting room with spacious 12 foot ceilings, a few chairs and a selection of magazines that date to the Eisenhower Administration. A bubbling fishtank containing a half-dozen bored guppies barely conceals the sound of the ventilation fan which cycles the air in the waiting room to the outside world once every 15 minutes.
Or, to describe the situation much less poetically, there's a single room connected to the outside environment via two flowpaths, an exhaust duct exiting through a 320 cfm exhaust fan, and an unfiltered air inlet. The room is 20 feet by 20 feet with a 12 foot ceiling (3.7 meters), giving it a free volume of 4800 cubic feet or about 135.9 cubic meters. The floor area is 400 square feet or 37.16 square meters; we will take this as the aerosol sedimentation area of the room. The intake and exhaust vents each have a flow area of 0.25 square meters and are located in the ceiling at an elevation of 3.7 meters. The ventilation system delivers 4 air changes per hour (ACH) which is (4 * 4800) / 60 = 320 cfm or 0.1501 cubic meters per second. We set both the supply and exhaust flowrates to that flowrate to keep pressure constant in the room. For simplicity, we ignore frictional loss in the duct and set the loss coefficent (CJN) to 2.78 which is equivalent to a sharp-edged orifice. CJN isn't important here due to the constant flowrate fan to but it's vital for modeling systems involving intercompartmental flow, natural circulation, etc. For modeling purposes, we assume that aerosols which exit the waiting room to the atmosphere cannot reenter.
In this analysis, the fishtank is not modeled.
Modeling the Aerosol Source
Our poor infected patient (Marty Measles) has a 100F-degree fever and is trying to hold himself together. He coughs once every five minutes (12 per hour) and each cough contains 6.0E-8 milliliters of virus-laden aerosol with a droplet size <= 10 microns. Compare this to a coughing rate of 3-24 per hour for pulmonary tuberculosis and 12-24 per hour for pneumonia. Multiplying the aerosol volume by the cough rate, we get a volumetric aerosol source rate of 2.0E-16 cubic meters per second.
Aerosol transport and deposition are a function of droplet size and aerosols tend towards a lognormal size distribution. To properly model the aerosol source, we need an estimate of the cough droplets geometric mean and geometric standard deviation. Conveniently, 3M Technical Bulletin Number 174 "Respiratory Protection for Airborne Exposures to Biohazards" provides a particle size distribution for sneeze droplets from which we can derive the geometric mean and standard deviation (1.5051 microns and 2.01, respectively). For the purpose of this analysis, we assume the sneeze droplets have the same physical properties as water (density, viscosity, surface tension, etc.)
This is all the information we need to describe Marty Measles as a continuous aerosol pathogen source. He has other redeeming qualities but they aren't relevant to this analysis.
Modeling Pathogen Uptake
Across the room sits Fiona Fitfiddle who has picked possibly the worst time to schedule a physical (she has an upcoming triathlon). Her breathing rate is typical for her age, about 0.4 cubic meters per hour or 1.111E-4 cubic meters per second. Inhalation dose is given by breathing rate times exposure duration times deposition fraction times airborne pathogen concentration. For 1 micron particles, the deposition fractions for the naso-pharynx, bronchial tree/windpipe, and pulmonary/lungs are 0.3, 0.08, and 0.25 respectively. These figures are consistent with the ICRP 30 model used for estimating radiation dose from inhaled radioactive particles and should be valid for aerosol pathogens.
From the CDC's information about measles transmission, we see that measles tends to take hold in the nose and throat so we use the naso-pharynx retention fraction of 0.30. Deposition in the nose and throat can be modeled as a HEPA filter with a decontamination factor of 1/(1 - f) = 1 / 0.7 = 1.43 where f is the filter efficiency or deposition fraction. We assume the pulmonary system has a volume of 0.05 cubic meters. This is higher than the tidal volume of 0.5 liters and the total volume of 6 liters, but it's irrelevant since deposition is only occurring in the filtered junction (naso-pharynx), not in the lung volume. Breathing rate determines deposition, not lung capacity. This gives us enough information to model naso-pharyngeal retention of an aerosol in Fiona's nose.
This completes the development of the air flow and aerosol deposition model used by FATE; the source, control volume, and flow path topology for this model is shown in Figure 1.
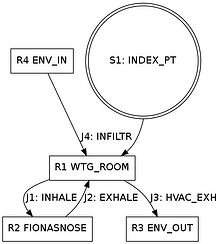
Figure 1: Ventilation Topology
Estimating Probability of Infection
Here we make several key assumptions. First, we assume that there is one measles virus per aerosol droplet, we count droplets (and thus viruses) by dividing the mass of deposited aerosol by the mass of a typical droplet. The aerosol geometric mean diameter is 1.5051 micron giving a droplet mass of 1.7852E-15 kg. In the case of pathogens which need only one virus or bacillus to cause infection (a conservative assumption), the probability of infection is (1 - exp(-n_virus)) where n_virus is the equivalent number of droplets (viruses) retained in Fiona's nose. This assumes a Poisson distribution for infection probability based on the Wells-Riley model of airborne disease transmission and that n_virus is greater than or equal to zero. The parameter n_virus is just m_retained / m_droplet so the probability of infection is (1 - exp(-m_retained/m_droplet)). Note that n_virus may be less than one.
Scenario 1: 30 Minutes of Source, 60 Minutes of Exposure
In the first scenario, assume Fiona and Marty both arrive at Dr. Bob's office at 8am. Marty has an 8:30am appointment and (being extra-punctual) Fiona has a 9am appointment. At the end of an hour, Fiona has 7.50E-17 kg of aerosol deposited in her nose and throat. Assuming 1.5 micron droplets, this means she's inhaled 7.50E-17 / 1.7852E-15 = 0.0042 droplets, giving her a probability of infection of (1 - exp(-0.0042)) = 4.1%.
Scenario 2: 30 Minutes of Source, 15 Minutes of Exposure
Let's change the scenario so Not-a-Doctor Bob can see Fiona after only fifteen minutes of waiting. In this case, Fiona has 1.46E-17 kg of aerosol deposited in her nose and throat. Using the same droplet size, she has now inhaled 1.46E-17 / 1.7852E-15 = 8.19E-3 droplets, giving her = 0.82% chance of infection.
Scenario 3: 30 Minutes of Source, No Ventilation
Overnight, the neighborhood squirrels have gnawed through the belt connecting the ventilation fan to its motor and Not-a-Doctor Bob hasn't noticed. Marty and Fiona arrive as before and note that the waiting room is stuffier than usual. Without room ventilation, Fiona's 15 minute aerosol uptake is 1.99E-17 kg, corresponding to 0.0111 droplets and a 1.1% chance of infection. Her 60 minute aerosol uptake is 2.39E-16 kg, corresponding to 0.134 droplets and a 12.5% chance of infection.
Scenario 4: 30 Minutes of Source, 3x Ventilation
Here Not-a-Doctor Bob has upgraded the clinic's ventilation system and replaced the old noisy 320 cfm fan with a beefier, quieter 960 cfm fan which now delivers 12 air changes per hour. Fiona takes in 9.06E-18 kg of aerosol corresponding to 5.07E-3 droplets and a 0.51% chance of infection after 15 minutes; after an hour she's taken in 2.66E-17 kg of aerosol, corresponding to 0.0149 droplets and a 1.48% chance of infection.
Scenario 5: 30 Minutes of Source, 1x Ventilation, 70% Efficent Mask
In our final scenario, Fiona has been paying attention to news stories about recent epidemics of vaccine-preventable diseases like measles and whooping cough. She's up-to-date on her vaccinations but has taken the extra precaution of wearing a NIOSH-approved respirator to Not-a-Doctor Bob's clinic. Unfortunately, her mask is not properly fitted so it only provides 70% filtration efficiency instead of its 95% efficiency rating. Also, Not-a-Doctor Bob has decided to save on power by turning down the waiting room ventilation back to its original 320 cfm.
Here we have adjust our model to add a new volume, a new flow path, and rearrange some other flow paths in order to model Fiona's mask. We duplicate the volume we use for modeling Fiona's nose to create a volume representing the gas space between the mask and her face; the volume isn't important, it just needs to be large enough to avoid causing the numerical solution of the problem from becoming too stiff but small enough that aerosol dilution isn't an issue. We then clone the filtered flow path representing the naso-pharynx deposition location and change the decontamination factor to 1/(1 - 0.7) = 3.3333 to represent the masks' 70% filtration efficiency. Finally align the ventilation path to go from the waiting room through the mask filter to the mask volume through Fiona's nose and back out to the waiting room. The revised flow topology is shown in Figure 2.
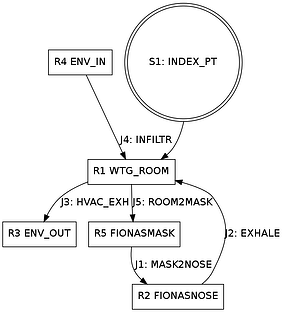
Figure 2: Ventilation Topology with Mask
The result is that Fiona's 15 minute aerosol uptake is 2.01E-18 kg or 1.12E-3 droplets, corresponding to an infection probability of 0.11%. After an hour, her aerosol uptake is 2.12E-017 kg or 0.0119 droplets, giving an infection probability of 1.18%.
Observations and Discussion
Figures 3 and 4 summarize Fiona's infection probability after 15 minutes and 60 minutes of exposure, respectively. The model results show what we intuitively expect: reducing exposure time, increasing room ventilation, and using personal protective equipment (PPE) all significantly reduce risk of infection due to aerosol pathogens. Figure 5 shows infection probability vs exposure time for all combinations of ventilation rate and mask use. To verify the aerosols are behaving as we expect, Figure 6 shows a mass distribution of the aerosol compared with its production. This shows expected linear production and exponential depletion behavior of the aerosol with time.
Figure 3: Infection Probability after 60 Minutes of Exposure
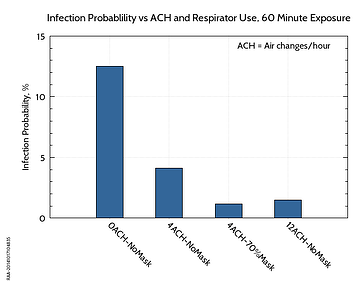
Figure 4: Infection Probability after 15 Minutes of Exposure
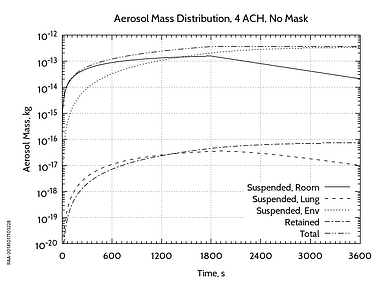
Figure 5: Infection Probability vs Exposure Duration and PPE Use

Figure 6: Aerosol Mass Distribution for Scenarios 1 and 2
Personally, I'm most surprised that despite very rough estimates of many of the parameters in this model, the infection probabilities seem fairly reasonable ranging from 0.1% to 13%. This is not a "cooked" analysis; none of the values were chosen to produce a reasonable-looking result. Rather, parameters were taken from a number of disparate sources - respirator selection guidelines, hospital isolation room ventilation standards, radiological analysis textbooks - and treated in a very ad hoc and mechanistic manner. Even so, the insight we gain is not from the raw numbers themselves but how they vary when we change aspects of the model. The model responds in a way we expect and generally confirms what we already know about ventilation, filtration, and airborne pathogens. This provides us with a firm basis to refine the model to a point where we can predict infection probabilities with an acceptable level of accuracy and confidence.
Limitations of this Model
As noted at the outset, this is a simplistic ad hoc model with known limitations. Here are a few I have identified; there are likely many more:
We explicitly ignore distance effects between pathogen source and receptor; Fiona is 2-3 times more likely to be infected if she is within 3 feet of Marty when he coughs.
We assume the droplet size distribution for a sneeze is a reasonable approximation of that from a cough.
We use a single retention fraction and filtration efficiency for all droplet sizes. Is this simplification reasonable for this sort of analysis?
We chose the ICRP 30 breathing model because it was familiar; is this model appropriate for simulating biological hazards? It would appear so since aerosol transport and retention should be independent of the contents of the aerosol. However different standards bodies may use different models so in an actual analysis we would use a model recommended by the applicable regulator or standards body. For the purpose of illustration, the ICRP 30 model is a reasonable initial choice.
We explicitly ignore sedimentation (gravitational settling) and turbulent deposition of the aerosol on walls and floors. Turbulent deposition on the walls may play a role but aerosols on the order of a micron tend to remain airborne rather than settling out by gravitation.
Note here, that if we ignore aerosol-specific phenomena and instead treat the aerosol as a generic airborne contaminant, there is a simple closed-form solution for pathogen concentration and biological uptake, making special-purpose analysis software unnecessary. However, if we don't know at the outset how the aerosol behaves regarding settling and deposition, we need tools sophisticated enough to model aerosol behavior to show which phenomena matter and which don't. In any case, it's important that tools serve the analysis, not drive it. Here the simplicity of FATE input, fast runtime, and straightforward presentation of results allow a variety of scenarios could be quickly analyzed once the initial model was built.
The ventilation rate has no firm basis. In an actual analysis, this figure would be supplied, either from design or measured data or as part of an upset scenario (e.g. ventilation failure). Despite being arbitratily chosen, the ventilation rate in this model is similar to what is recommended in standards for hospital isolation ward ventilation design.
We estimate the pathogen concentration in the aerosol. For the purposes of illustration, assuming one virus per 1.5 micron droplet may be appropriate but in an actual case, we would need a much stronger basis for this concentration. The current model provides no basis for the assumed pathogen concentration.
We assume only one virus or bacterium is necessary to cause infection. For measles, this is not a bad assumption since we know empirically that measles is an extremely virulent disease. This assumption is conservative and justifiable but may warrant review for a best- or nominal-case analysis. Also, while this assumption may be reasonable for especially virulent diseases, it may not be appropriate for others (e.g. Ebola).
We assume our use of the Wells-Riley model is correctly implemented, appropriate, and accurate. I have no specific expertise in airborne pathogen modeling or with the Wells-Riley model in particular. However, I have seen this formulation referenced in NIOSH documents on respirator selection for biohazard protection and I'm taking it on faith I'm applying it correctly. Again, for the purpose of illustration this may be appropriate, but in an actual analysis the choice and implementation of this model would need much stronger justification. This is where the combined efforts of engineers and health professionals are needed.
We provide no uncertainty ranges. This is particularly important in probabilistic models where uncertainty in input values can be large enough to make quantitative predictions useless. In an actual analysis, most of these limitations could be addressed with better data sources and literature references, others might be addressed by adding safety factors or similar conservative engineering techniques, and some may just have to be acknowledged. No matter how they are dispositioned, it is important they are clearly stated so the reader can set their confidence in the model accordingly.
To that end, there is a practice in radiological analysis to refrain from presenting results which are highly uncertain or are based on incomplete data or simplistic models to avoid two serious problems in risk communication: giving a false sense of security, and inciting panic by overestimating risk. This is a particularly acute problem when discussing radiological risk but it is also applicable to discussions of biological risks such as infectious diseases. The disclaimers and discussion of limitations above are not defensive legalese; they are a very important part of responsible risk communications.
Conclusion and Afterword
Hopefully, you've enjoyed this excursion into the world of aerosol modeling and biological hazard analysis. While this blog-essay was intended as a demonstration of FATE's modeling capabilities outside its typical problem domain of nuclear safety analysis, it is more illustrative of the process of engineering analysis and modeling that Fauske & Associates can bring to bear on a problem, the insight which can be gained from facility modeling, and the recognition of the limits of a model's applicability and accuracy.
Please contact us to discuss Fate™, modeling, hazards analysis and other nuclear safety concerns at info@fauske.com